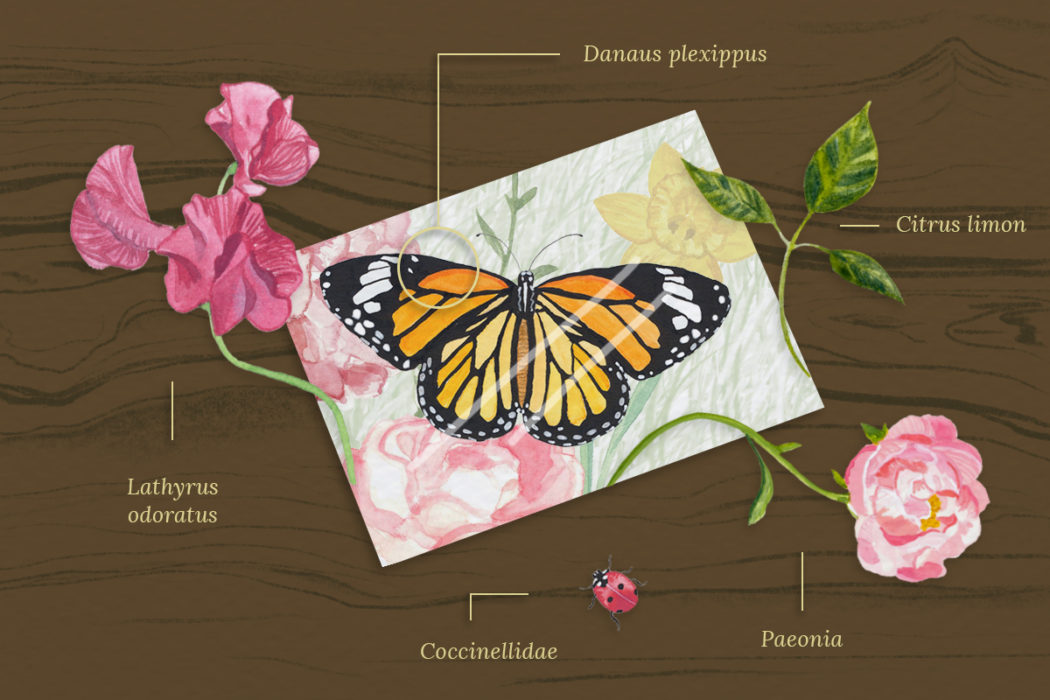
To gauge an ecosystem’s health, one can look to butterflies. Fortunately, because they are quite beautiful, we’ve been looking at them for a long time. We know these technicolor insects thrive in habitat that is typically well-suited to a host of other bugs — plus the birds and bats that feed on them, and so forth up the food chain. Accordingly, butterfly counts serve as important proxies in studies of conservation, migration, biomimicry, and climate change.
Just as they painted and preserved butterflies in earlier times, naturalists today capture them with smartphone cameras. For University of Arizona wildlife biologist Katy Prudic, those photos are a treasure trove. Prudic helped create eButterfly, a platform on which butterfly enthusiasts have shared photos of 1,038 species. The photos aren’t just lovely — they tell scientists like Prudic where and when a butterfly was found.
“That data can then be used to answer a variety of scientific questions, many of them trying to figure out what the impacts of climate change are doing to our little pollinator friends,” Prudic said. By comparing a species’ historical range with the locations modern photographers are seeing them, Prudic can glean how certain species’ ranges are shifting, and better guess where they’ll be 20, 50, 100 years from now. Conservation measures, like meadow restoration, can be planned accordingly.
But that’s just the start. Throw in artificial intelligence, and scientists can map out far more complex ecological relationships. For instance, a photo on eButterfly might have multiple plant species in the frame. It’s conceivable that, in the near future, AI will be able to automatically identify those plants and associate them with certain butterfly species. “And then you can start almost building, for lack of a better term, a Netflix recommendation model,” Prudic told me.
Hurdles remain, but Prudic and other conservation scientists see a future in which data gathered from smartphones, drones, satellites, and remote sensors yield insights, shaped by artificial intelligence and machine learning, that can drive conservation in the digital age. It’s a welcome development at a time when climate change and habitat loss heighten the need for targeted measures to stave off extinction.
At this point, the main perk of artificial intelligence is alleviating scientists’ most time-consuming chores. Ned Horning, director of applied biodiversity informatics at the American Museum of Natural History, says that turning raw images or audio into useful data is an incredibly monotonous process that is subject to human error.
“If you take a picture of birds, some of these might have 10,000 birds in them. So you’ve got a researcher spending the better part of a day counting 10,000 birds,” Horning said. “A trained computer could do that in a minute.”
To that end, Horning helped start the Animal Detection Network, which produces open-source tools that label and count animals in images. The technology is still in its infancy, but Colorado Parks and Wildlife is already using a version of it to identify and count species photographed by camera traps.
The progress is valuable. Wildlife organizations that monitor camera traps can have stockpiles of millions of images, Horning said. If a well-trained AI can whittle that down to merely thousands of photos with a high probability of an animal actually being in the image, it saves researchers tons of work — and lessens the odds that a bleary-eyed grad student messes up a classification.
Drone photography is another technology boosting demand for conservation-capable AI. Researchers and conservation groups now use relatively inexpensive drones to snap hundreds of aerial landscape photos at a time. Horning said there’s good software available to stitch images into a single mosaic. “But then if you you want to extract data from those images” — say, identify tree species in a timber plot — “that’s where things get tricky, and the state of the art is lagging behind the interest of conservation practitioners,” he said.
In a recent study, Horning and a team from the University of California, Davis tested various AI programs’ abilities to identify cheatgrass, the fire-prone invasive that’s rampant across the West, in aerial images of Nevada’s sagebrush steppe. The team found that some programs worked reasonably well, but only under certain conditions. Mornings and evenings, for instance, were bad times for photos — shadows sometimes tricked the algorithms. And early spring was the best time to fly the drone, as cheatgrass greens up earlier than most native species.
AI’s potential extends well above the heights drones can reach. In 2000, NASA launched its MODIS satellites, some of the earliest reliable sources of Earth imagery captured from space. (The MODIS daily gallery is a treat.) That collection of images presented an opportunity for Jerod Merkle’s team, which studies animal migration at the University of Wyoming. With the MODIS imagery, “we can really drill down on a given spot on Earth for the last 20 years,” Merkle said. Coupling the satellite imagery with data from Yellowstone bison fitted with GPS collars, Merkle and his team found that the heavy grazing of bison herds re-starts plant growth, much like the onset of spring.
But as technology — AI included — improves, they’ll be able to learn much more. For one, Merkle is working with enormous data sets. GPS collars currently in use provide about 100,000 data points over their lifespans, so collaring 100 bison gives researchers 1 million points to sift through. And that total, as technology improves, will swell. Thanks to smartphones and smartwatches, body-monitoring hardware is getting better and smaller. If we’re constantly monitoring our own vital signs, Merkle figures, why can’t we do the same for bison? Couple a sensor-laden collar with solar-powered batteries, and one could ascertain incredibly precise measurements over the course of an animal’s life.
“And we can use machine learning to make sense of those data,” Merkle said. “Is the animal foraging? Is it sleeping? Is it running? By having this detailed extra data that’s more physiological, we’re going to be able to uncover the life of the animal.”
The barrier to such machine learning algorithms is the training they require. A program that can automatically distinguish between a bison that is foraging or sleeping or identify the checkermallow in the background of a butterfly image must first learn, via enormous datasets, how to make those distinctions. That requires meticulously labeled datasets, created by humans. Getting AI to save us work will require, at least up front, tremendous amounts of work.
But the need for such conservation assistance is increasingly clear. Despite the threats associated with climate change, global emissions continue to increase. Further, humanity continues to convert natural habitat into monoculture plantations, subdivisions, and other landscapes not suitable for butterfly, bison, or any other creature for that matter. That, Prudic said, makes the speed enabled by machine learning and AI critical.
“What we’d like to do is understand how the ranges of all 750 butterflies in North America have changed — where they have expanded or shrank or moved in the last 50 years. And then, hopefully, we can build models that predict where they might be in the next 50 years,” Prudic said. “But because of the scale of the data we have, we have to build in some pretty fancy research computing infrastructure.”
Our planet is changing at an unprecedented pace. Perhaps AI will allow conservation to keep up.